Current Projects
Industrial Assessment Center (IAC)
Students: Deokgeun Park, Shambhavi Singh, Rafael Dugarte, Taeksoo Kim
The Texas A&M University (TAMU) Industrial Assessment Center (IAC) proposes to train/employ 12 young engineers (engineering undergraduate and graduate students) annually and conduct 24 assessment days in 22 small and medium-sized manufacturing plants each contract year. The engineering students will receive hands-on and formal training about industrial energy conservation, waste reduction, and productivity improvement. Within 60 days of each assessment visit, a formal, technical report will be supplied for each plant, and assessment data will be uploaded to the national IAC database. Twenty-four assessment days in 22 plants allow two plants each year to have two-day assessment visits due to facility size and/or complexity, and in coordination with the state Manufacturing Extension Partnership (MEP). In the event two plants are not identified for two-day assessment visits, more plants will be selected so that the contracted number, 24, of assessment days is fulfilled. Additional multiple-day assessments will be with DOE approval, to serve plants with relatively large energy bills, or to serve large, complex plants or processes.
Peak Electrical Demand Reduction in Fluid Distribution Systems through Load-shifting using Model Predictive Control
Students: Rafael Dugarte
The purpose of this project is to demonstrate potential cost-saving control strategies to be applied in the areas of wastewater treatment through peak electrical demand reduction. Peak demand is reduced by actively modulating the various system pumps and using the excess tank volume as a virtual “battery” to forcibly reduce periods of high electrical demand through 15-minute averages.
Large Scale Data Analytics for Fault Detection and Diagnosis of Residential HVAC Systems (Sponsored by Trane Technologies Inc.)
Students: Fangzhou Guo
HVAC systems are prone to faults that degrade system performance, causing extra energy consumption and occupant discomfort. Fault detection and diagnosis (FDD) assists in identifying specific system faults, predicting gradual degradation, and prompting necessary maintenance. However, for residential HVAC systems, the FDD methods are relatively limited, mainly because FDD requires the installation of additional sensors on each HVAC equipment, which is not cost-effective for the mass-produced residential systems. An alternative data source is smart thermostat data, which records important, but limited information.
Trane Technologies Inc. provides a cloud-based dataset that contains thermostat history records of nearly 560,000 distinct residential HVAC systems. Using this large, diverse, and growing dataset, this project develops novel statistics-based and artificial intelligence-based methods for detecting and diagnosing faults from a large number of residential systems.
Large Scale Data Analytics for Residential HVAC Systems using Connected Smart Thermostat Data (Sponsored by Trane Technologies Inc.)
Students: Arvind Vadali Phani
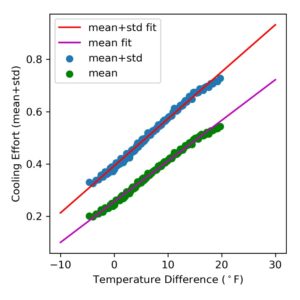
Regression model showing the performance of mean system and the mean +1std system of a dataset.
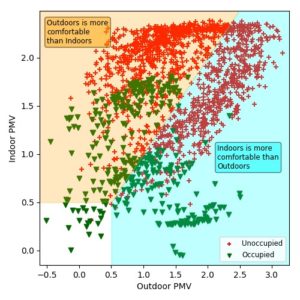
2-hour resampled data from a system showing the thermal comfort value of the indoors in comparison to outdoors.
My work on this project is mainly in two avenues. I am working on building metrics to characterize the impact of residential HVAC systems on occupant’s thermal comfort and total energy savings using smart thermostat data. Subsequently, the metrics are then being used to develop a score to measure the severity of the impact they have on the occupant, using which the most severe systems would be labeled for repair. The second topic I am working on is to characterize the relative influence of various factors that affect the thermal response of a house. The objective of this study is to filter data from houses with faulty HVAC systems with data from systems built in houses with poor building energy characteristics.
Solar Photovoltaic Systems: Forecasting, Tracking, Sizing and Optimal Scheduling
Students: Hussein Sharadga
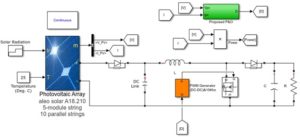
PV System power control.
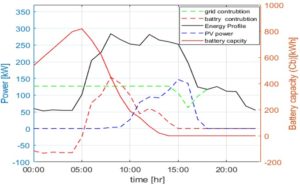
Peak shaving.
The project aims to design a solar PV-Battery system used to reduce the bill amount and to secure the grid operation. The grid is secured by shaving the peak and by reducing the excess PV energy. The work aims to develop a framework used to construct an energy schedule. The efficient secluding requires a reliable forecasting tool. The modern neural network configurations and the well-known statistical approaches are applied. The forecasting tools cannot be 100 % accurate, thus uncertainty needs to be included in the decision making. Stochastic dynamic programming is the most efficient tool used for energy planning under the Load and PV uncertainties. Before constructing the energy schedule, the system components, i.e., its capacity needs to be optimized. Most of the PV systems are integrated with a maximum power point tracking controller. However, the controller can be modified to make a balance between the PV energy, the demand, and the space available in the battery to prevent any excess PV production.
Intelligent Storage System for Industries with Intermittent Demand of Compressed Air
Students: Taeksoo Kim
Schematic of the storage system.
Storage tanks and valves used in the experiments.
A tremendous amount of energy (about 900 million megawatthours (MWh)) is consumed every year in manufacturing industries. Approximately 10% of it is used for generating compressed air according to the Department of Energy. Thus, reducing energy consumption while ensuring the performance of compressed air systems is important.
This research aims to develop an intelligent storage system for compressed air lines with intermittent demand. The proposed design of the system will be able to adapt to the varying demand requirements while reducing the operation time of the compressors. As a result, there will be lower energy consumption and lower costs for running compressed air systems. The system will first experiment at a laboratory-sized system, which will later be implemented for industrial application.
Aviation-class Synergistically Cooled Electric-motors with Integrated Drives (ASCEND) (Sponsored by U.S. Department of Energy and ARPA-E)
Students: Deokgeun Park
Test fixture for cooling systems.
Logo of ASCEND
Design, fabrication, and testing of a lightweight and ultra-efficient electric powertrain for aircraft propulsion to reduce the energy costs and emissions of aviation. I, as a part of a multidisciplinary team, will explore single and two-phase thermal management options with zeolite thermal energy storage to absorb the excess heat generated during takeoff.
Past Projects
Enhancing Energy Efficiency of Buildings using Smart Technologies and Automation
Students: Saurabh Shekhadar
Steady-state Thermal Modeling & Value Analysis of Commercial Chiller System With Oil-free VS Centrifugal Compressor (Sponsored by Emerson Commercial & Residential Solutions Inc.)
Students: Deokgeun Park
Distributed Model Predictive Control for Building Energy Systems (Sponsored by National Institute of Science and Technology)
Students: Matthew Elliot, Chris Bay, Rawand Jalal, Rohit Chintala
Heat Pump Dynamic Simulation Model (Sponsored by Emerson Inc.)
Students: Shuangshuang Liang, Chao Wang
National Science Foundation Career Award
Students: Shuangshuang Liang, Matthew Elliot